“Ridon Vehicle” paper accepted
SAE MobilityForward Challenge: AI Mini-Challenge
SAE MobilityForward Challenge: AI Mini-Challenge sponsored by Ford Motor Company was held on October 25-26, 2021.
Congrats to Assistant Professor Jaerock Kwon and students Huda Hussaini, Mintu Mariya Joy, Richa Chachra and Shouryan Nikam — members of a UM-Dearborn team that racked up several third place finishes in a recent SAE Mobility Forward AI Mini Challenge. The competition challenges universities to apply novel data science techniques to emerging technology issues relevant to the mobility industry. The UM-Dearborn team’s project identified socio-economic disparities in transportation for Pennsylvania residents and outlined options for innovative services that could make transportation access more equitable.
UM-Dearborn’s Mauto team won 3rd place in all three categories. I am very honored to be a faculty advisor of the team.
Awards
- Solutions Stakeholders Report – 3rd place – $100 – University of Michigan – Dearborn
- Stakeholder Presentation – 3rd place – $100 – University of Michigan – Dearborn
- Showcase Booth – 3rd place – $100 – University of Michigan – Dearborn
Team members
- Members: Huda Hussaini, Mintu Mariya Joy, Richa Chachra, Shouryan Nikam
- Mentors: Jaerock Kwon, Songan Zhang (Ford), Eric Tseng (Ford)
OmO: One-minute Only, all you need.
- Source Code: https://github.com/jrkwon/oscar
- Data location: https://drive.google.com/drive/folders/1jzhYka9HaKet8SCMlTGZOCRoWol8M2IW?usp=sharing
- Paper: TBA
OmO is an incremental end-to-end learning for lateral control in autonomous driving.
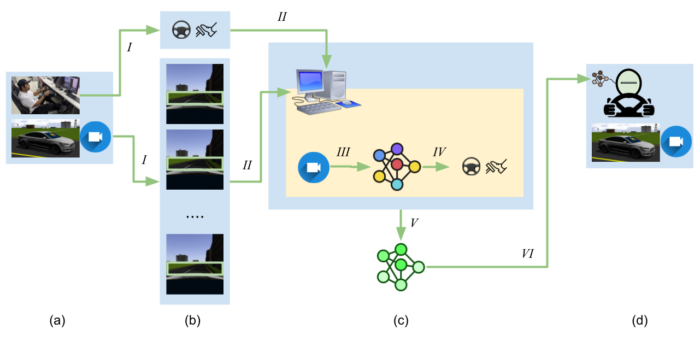
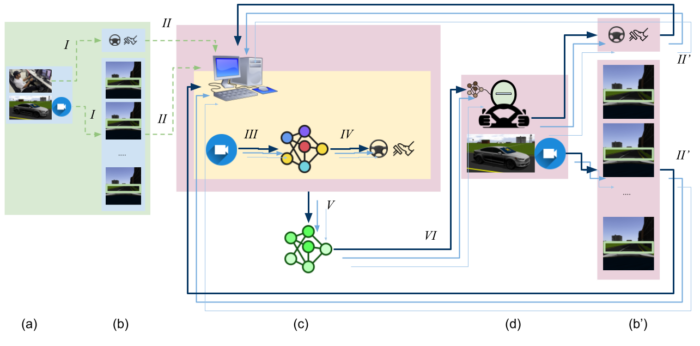
Abstract
Developing an autonomous driving system necessitates the use of high-quality data. However, due to the high cost of human labor, collecting driving data is often too expensive. With minimal initial data from a human driver, this research provides a novel strategy that uses an incremental approach to data collecting and neural network training. The proposed method, One Minute Only (OmO), is an end-to-end behavior cloning methodology that uses a convolutional neural network to develop a lateral controller for a vehicle. OmO begins by collecting the minimal amount of human driver’s driving data, which includes steering angles, throttles, velocities, and geographical locations. The human driving data is used to train a convolutional neural network. The trained neural network is then deployed to the vehicle’s driving controller, an Artificial Intelligence (AI) chauffeur’s brain. A human driver is no longer in the loop at this stage, and the inexperienced AI chauffeur drives the vehicle on a simulated track to collect some further data. The collected driving data will be fed into a convolutional neural network training module, which will help the AI chauffeur develop a new and, hopefully, stronger neural controller. The two steps of data collection and neural network training with the gathered data alternate until the neural network learns to properly correlate an image input with a steering angle. Extensive experiments have validated our proposed work, OmO. We anticipate that by utilizing this incremental driving data collection and neural network controller training, human effort and time will be considerably reduced, and the development of autonomous car technology will be accelerated. The findings of the experiments, as well as data and other material, are available online.
Introduction
Data collection needs huge human effort. In this paper, we provide a novel strategy in which a human driver is required to drive only as much as is necessary, and an Artificial Intelligence (AI) chauffeur powered by a deep convolutional neural network drives on behalf of the human driver when more driving data is required. Figure 1 shows the high-level system overview. To begin, a human driver drives a virtual car and captures only the bare minimum of data needed to train a neural network to drive a short distance by replicating the human driver’s behavior. A human driver is no longer in the loop at this time. After that, a driving controller employs the trained neural network. We gave it the name of an AI chauffeur who is driving a simulated vehicle. We recorded driving data for a little longer as the AI chauffeur drove the vehicle. After training a new neural network with the newly obtained data, the AI’s brain is transplanted with a new neural network in the hopes of improving the AI’s driving performance next time. This process may be repeated iteratively until we have a neural network that can reliably predict steering angles enough to control the lateral motions of the simulated vehicle.
Method
To implement and test our approach, we have been developing an open-source platform, OSCAR (Open-Source robotic Car Architecture for Research and education). https://github.com/jrkwon/oscar
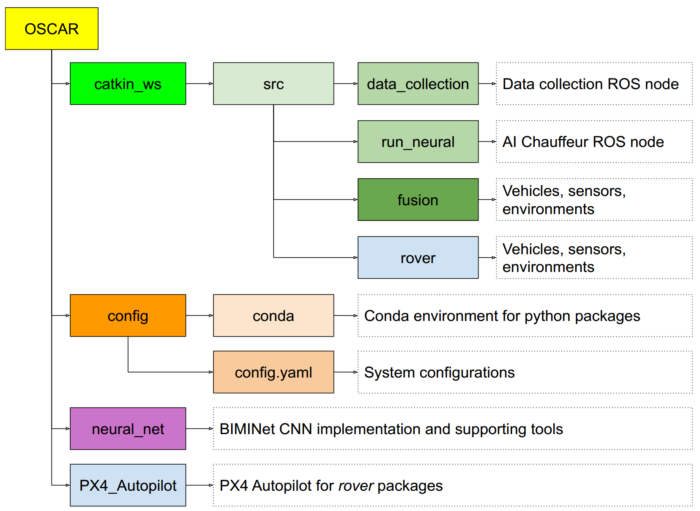
Vehicle Design
The chassis of the car is based on the Ford Fusion model. Three cameras are mounted on the front windshield. In this article, we solely used the front camera. Ouster’s 64 channel 3D LiDAR [11] is mounted to the top of the windshield. We did not employ the LiDAR sensor for this paper. The simulated car is built as a plugin within the fusion ROS package.
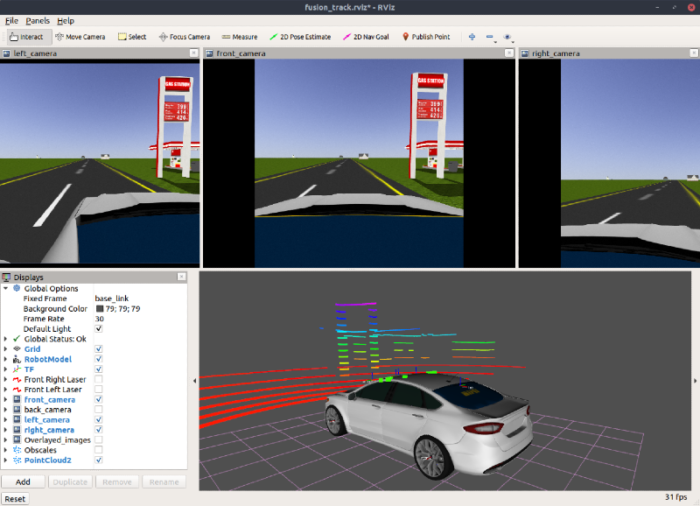
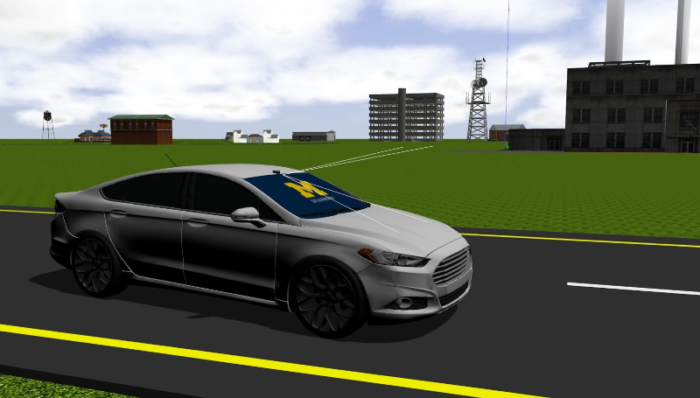
Tracks Design
We designed two different tracks in the Gazebo world format (Track A and B). Track A was utilized to collect data for neural network training. The original track design came from Dataspeed ADAS kit Gazebo/ROS simulator, which includes a track for a lane-keeping demonstration. The track is created with modular road segment models that include straight road segments of 50, 100, and 200 meters and radius curve road portions of 50 and 100 meters. We embellished the track with roadside objects such as gas stations, residences, and major architectural complexes. This is important to give some variations in the roadsides to make the lane-keeping task more practical and realistic. In this work, all data collection tasks were conducted for training in Track A.
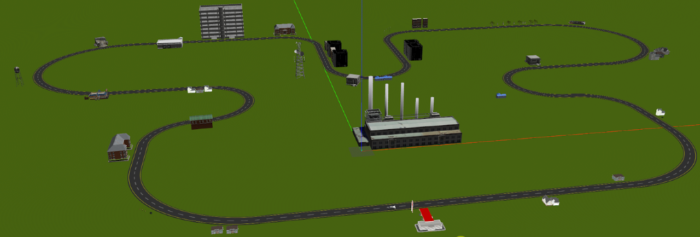
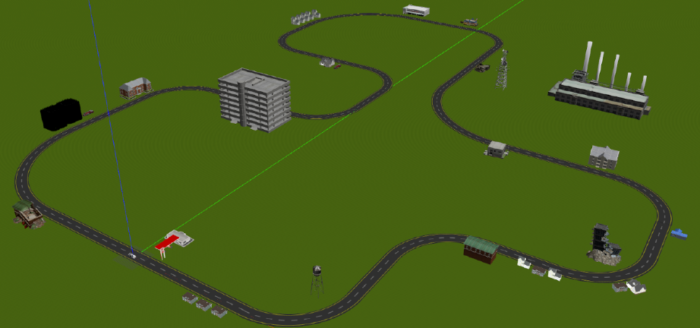
Data Collection
A simulated vehicle in the OSCAR platform sends its current velocity and position in a ROS topic named /base_pose_ground_truth. The current steering angle and throttle position of the vehicle is being sent through a ROS topic named /funsion. The front camera is used to collect data in this paper. The topic name of the camera image is /fusion/front_camera/image_raw. The image message must be converted to be saved as an image file. This can be done by cv_bridge.
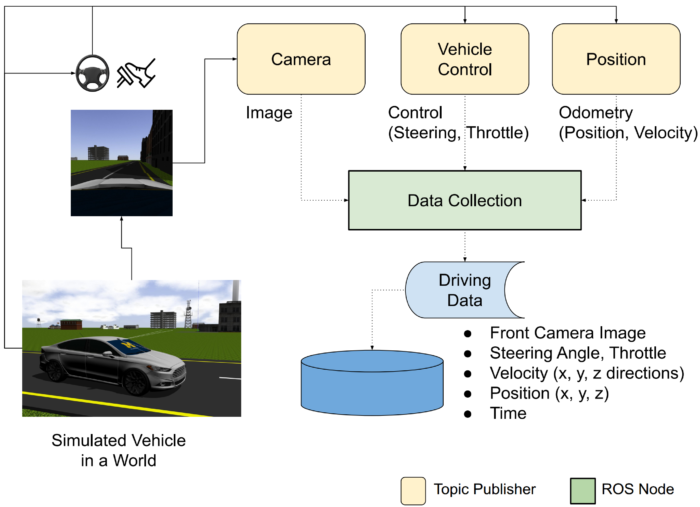
Training
The image below is the neural network architecture that we used.
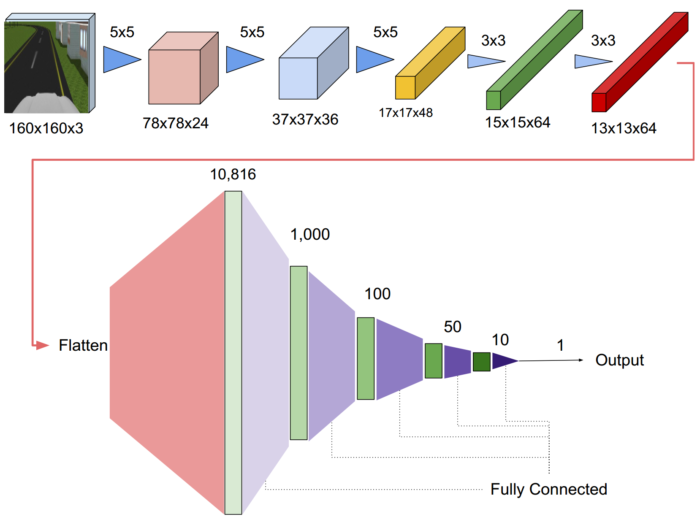
The training process can be described as follows.
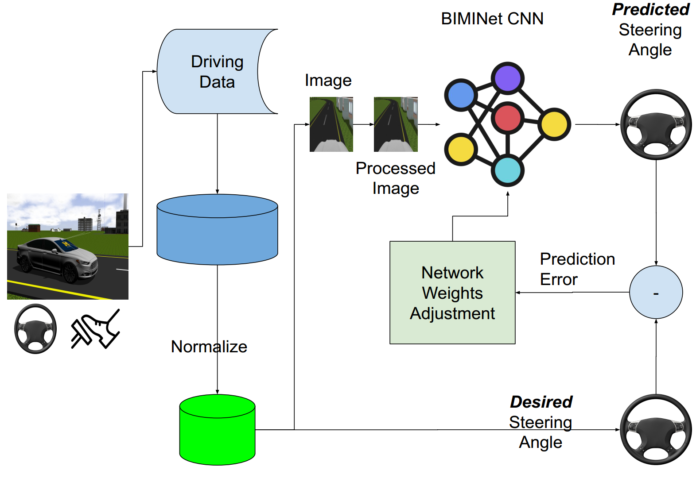
Experimental Results
We collected one-minute driving data from a human driver and used the data to train a neural network. The first trained neural network will be deployed to an AI chauffeur who is like an inexperienced driver. We have the AI chauffeur drive the vehicle to collect the 2nd round of driving data. The collected data from the AI chauffeur who is not good at driving will be fed to train the next level of training. We continued this data collection and training cycle until the trained neural network successfully drives the track.
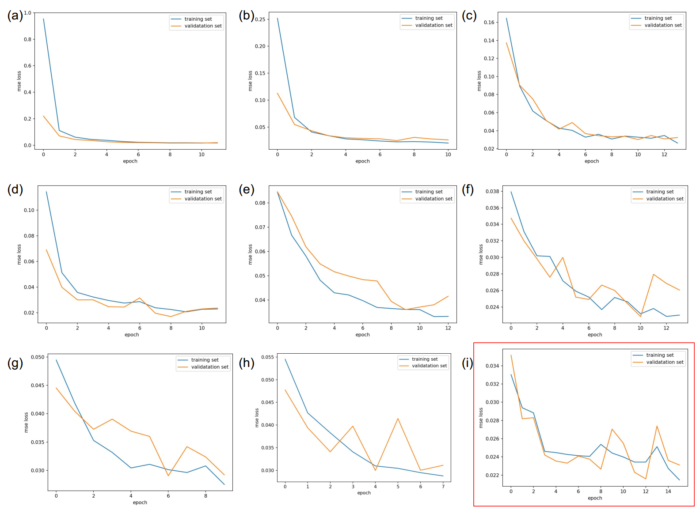
All data collected are available at this link. (TODO: add the Google Drive link of the datasets)
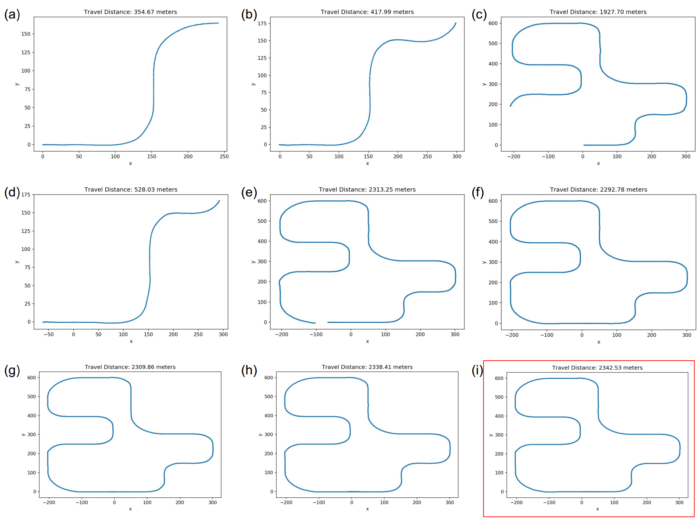
Data Visualization
Discussion
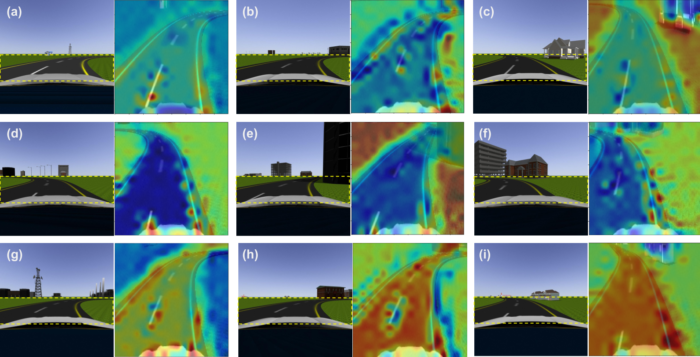
Activation maps. (a) – (i) are one minute, one minute 42 seconds, two through eight minutes respectively. Left in each label is a camera image input and right is an overlaid activation map on the region of interest. Blue is low and red is high activation values. The activations are taken from the last convolutional layer in the network. (a) show low confidence overall but some activations on the edges of the road. Interestingly up to five-minute data (d)-(f), neural networks learned the shapes of the roads is more important than the road itself. This can be a reasonable choice for neural networks to minimize MSEs of predictions due to the lack of varieties of both sides of the road. As more datasets were fed to the neural network training (g)-(i), the neural networks have to change the strategy to minimize MSEs due to different objects residing on the left and/or right side of the road that are not supposed to affect the steering angle predictions.
Conclusion
We offer OmO, a unique method that uses an incremental approach to data collection and neural network training using minimal data from a human driver. Extensive testing using two separate tracks and two different driving circumstances successfully validated the proposed method. We also developed OSCAR, an open-source platform for autonomous driving. We anticipate that by utilizing this incremental driving data collection and neural network controller training with the open-source platform, OSCAR, significant human effort and time will be saved, hence expediting the development process of autonomous car technology. As future work, we are planning to automate the entire process except for the initial human driving data.
Job Posting
Prof. Kwon is looking for an MS student for conducting research on human motion inference. Read the following page for more details.
Send an email to jrkwon@umich.edu stating your research interest with your CV. Knowledge of machine learning and data analysis is required.
- Application deadline: Oct 31, 2021
- Appointment type: hourly based for 2 to 3 months
New Bimians
We have new lab members. Welcome aboard!
Andrew Soong, M.S. student in Mechanical Engineering, Ann Arbor Aws Khalil, Ph.D. student in Electrical and Computer Engineering Jesudara Omidokun, M.S. student in Electrical and Computer Engineering
Joint Conference on AI in Smart Cities
On April 7, the Joint Conference on AI in Smart Cities hosted by KSCEE, KOTAA, and KOCSEA was successfully held in virtual.
- KSCEE: the Korea-American Society of Civil and Environmental Engineers (KSCEE)
- KOTAA: Korean Transportation Association in America (KOTAA)
- KOCSEA: the Korean Computer Scientists and Engineers Association of America
As conference co-char and president of KOCSEA, I am so happy that there was no technical difficulties during the conference. I also belive that parcipants like the conference.




Job Posting for 2021 SURE
Objective:
The Bio-Inspired Machine Intelligence (BIMI) lab (https://bimi.jrkwon.com) is looking for one undergraduate student who is interested in research funded by the 2021 Summer Undergraduate Research Experience (SURE) program.
Research Summary:
Driver Style Transfer for Autonomous Driving
People drive a vehicle differently. Yet, self-driving has a pre-defined style designed by manufacturers. It can be very uncomfortable for a driver who is aggressive in driving when a self-driving vehicle drives very cautiously. This can also be true vise versa.
To provide an individualized autonomous driving experience, we will collect several driving styles from human drivers in a simulated environment. Then we will extract features from the driving data that can represent driving styles.
To validate our approach, we will create a driving model from human driving data and the model will be deployed to a controller system of a simulated autonomous vehicle.
Through this research, we will be able to provide an individualized autonomous driving experience for a driver who can feel the autonomous vehicle drives just like how the driver usually drives.
Responsibilities:
- Read papers to understand the problems.
- Participate in data collection, processing, and management.
- Implement a neural network using Keras and/or PyTorch.
- Prepare technical report.
Qualifications:
- Required experiences:
- Python Programming skill
- Literacy in Linux commands
- Machine learning toolkit: Keras and/or PyTorch
- Preferred
- Git and GitHub(or GitLab)
- Robotic Operating Systems (ROS)
- scikit-learn
- pandas
Program Requirements:
- The student must be a registered UM-Dearborn undergraduate student with an expected graduation date no sooner than December 2021.
- The student will receive a stipend of $3,200 to be paid in installments throughout the project period (May-August). Students will receive payments of $1,000 the first week of June, July, and August, with a final payment of $200 after the Showcase in September.
- Note, if the student receives financial aid, they should check with the Financial Aid Office to determine if this stipend will impact their financial aid package.
- The student should not receive duplicate support while participating in this program; supplementing with external funding during the duration of the SURE program is NOT ALLOWED.
- Student participants and faculty mentors must attend the SURE Kickoff on Tuesday, May 18th at 4 pm.
- The student is required to attend the SURE Professional Development Seminars taking place on the following dates: 6/3, 6/17, 7/8, 7/22, 8/5, and 8/19 (all sessions run from 1:00 pm-2:00 pm; a schedule will be provided at the Kickoff event).
- The student is expected to present their work at the SURE Project Showcase in the fall.
Contact Information
Please contact Prof. Jaerock Kwon at jrkwon@umich.edu.
Data Viewer for OSCAR
I have just completed implementing a data viewer for the OSCAR (http://github.com/jrkwon/oscar).
It can show the labeled steering angles and corresponding predictions to visually perform a neural network’s inference.
Working during Pandemic
My office is closed and Robotics Lab is not allowed to be used for research. So, I have been working in a temporary space that is wide open in the IAVS. It is actually not bad to use a large space alone most of the time.
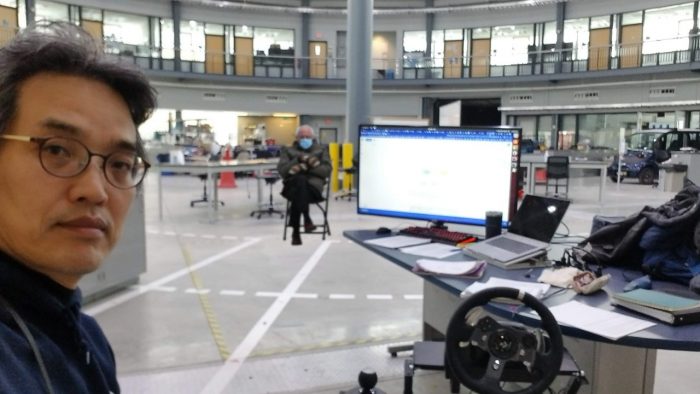